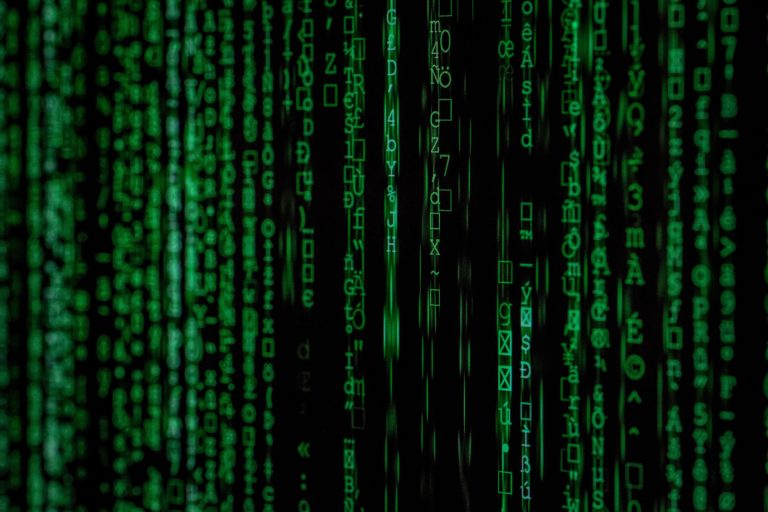
Hidden biases and mindful data
From Ottawa to Montreal
At LMIC, we see collaboration and information sharing as key ingredients to the success of what we do. This includes, among other things, understanding how better access to quality labour market information (LMI) can improve outcomes for underrepresented populations. Thus, when I learned that the Quebec Inter-University Centre for Social Statistics (QICSS) conference focused on immigration, I dusted off my French–English dictionary and packed my bag for Montréal.
Visions of datasets dance through my head
The conference, held at HEC Montréal, served as a forum for social scientists from Canada and the US to collaborate and share their current projects, which use the latest Canadian business and social data available. Tapping into new data sources such as the Canadian Employer–Employee Dynamics Database (CEEDD), as well as older ones like the Intergenerational Income Database (IID), the Workplace Employee Survey, and the Permanent Resident Landing File (PRLF), researchers are able to investigate such topics as the link between literacy and labour market imbalances by skill level, the relationship between immigration and productivity, and so on.
As an economist whose own work focuses on labour market information (LMI), I hoped that the research of my peers would help motivate a discussion on the role of LMI in explaining, and hopefully improving, the economic and social outcomes of immigrants in Canada. As every researcher knows, data is most commonly the limiting factor in any successful study, so the prospect of new or updated datasets offering better local, more granular information is exhilarating.
An unexpected takeaway
During the two-day conference, I listened to many fascinating presentations and studies; and although I came away as hoped—with ideas for how LMIC might support the measurement of labour market outcomes for underrepresented populations—it was an unexpected message by sociologist Richard Alba that really resonated within me.
Using data from the United States, Alba discussed Ethno-racial realignment in North American immigration societies. He explained that demographers commonly treat ethno-racial groups as discrete and distinct, especially for population projections. Research shows, however, that the boundaries between ethno-racial groups are blurring. Greater social mobility of minorities has resulted in greater integration, frequently including intermarriage with whites. Moreover, the children resulting from these intermarriages identify more commonly with their majority origin rather than as a minority. Because of the way the census is constructed, however, this fact is not captured in the data.
The message
Finding, accessing, and even using the right data can be challenging. On top of this, accessibility is often further limited by issues of confidentiality. Thus, it is important for the information that does exist to be as accurate and meaningful as possible. As researchers, we can get so accustomed to having to adjust for not being able to get the right data, that we forget to ask why it is so, assuming some greater good (e.g., confidentiality), or some other scientific imperative. But, as Alba reminds us, we must be mindful of how data is constructed in the first place.
According to Alba, when the US Census Bureau planned to introduce a multiple race option in 2000, civil rights organizations feared that African-Americans who indicated “mixed” as their racial identification would not be counted as Black and end up being “lost.” The Department of Justice consequently decreed that, for civil-rights purposes, mixed majority-minority individuals would be classified by their minority origin. The Census Bureau followed along. Alba’s research suggests that census data and projections, because of inadequacies in the classification of children of intermarriages — who now number about 15% among recent births – are increasingly diverging from on-the-ground social realities.
Takeaways
At the end of the conference, I reflected on Alba’s example and the lesson it contained. I was reminded of LMIC’s dedication to integrity and transparency. I thought about the importance of academic research and the role of good data in the decision-making process. I considered LMIC’s own commitment to objectivity and fairness. And I couldn’t help but think that we must all do our part to improve the quality of data by being mindful of how and why it was constructed in the first place. Does it really reflect how people see themselves, or does it reflect some institutional or government strategy?
Other presentations from the conference can be viewed and downloaded via the Conference Program.
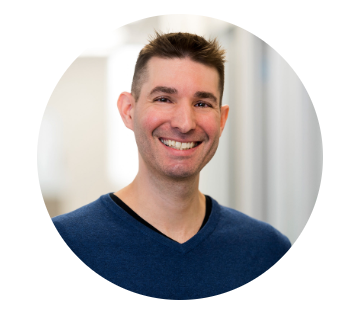
As an economist with LMIC, Anthony Mantione contributes to advancing LMIC’s mandate through the application of computational techniques to data analysis.