Table of Contents
Key Findings
- LMIC’s public opinion research on the labour market information needs of Canadians has highlighted the importance of information that is relevant to the decision at hand. For many Canadians this means information specific to their town or job and for others, including stakeholders, it means having information that is recent and up to date. Indeed, our partners and stakeholders have long called for more granular information at the local level to support better, more informed decisions — a point higlighted in our inaugural LMI Insights No. 1.
- It is with this in mind that LMIC and Statistics Canada, in collaboration with its stakeholders, have assessed several approaches to attaining more local (smaller area), granular (more detailed information), frequent (more often), and timely (more up to date) labour market information. The approaches evaluated and discussed in this Insight include 1) survey-based options, 2) linking administrative data, and 3) modelling methods.
- The three proposed approaches vary considerably in how they improve upon prevailing labour market information. No approach is a panacea, and each entail different trade-offs. More granular data may be feasible, but to the detriment of localness, for example. The three approaches also have very different cost implications.
- Based on our preliminary assessment, as a first step, LMIC and Statistics Canada will explore the feasibility of a specific modelling method called small area estimation (SAE). In this respect, the breadth of existing sources of labour market information — such as the Labour Force Survey, census data, and other datasets — are essential tools for leveraging this approach.
- After receiving feedback from our stakeholders and partners, we will re-evaluate how well this new information meets their needs. We will adjust the project as necessary, either to scale up the implementation of local, granular data generation or to revisit the approaches presented here.
Introduction
LMIC’s first LMI Insights documented the lack of local, granular, frequent, and timely data as an important gap in Canada’s labour market information (LMI) system. Recognizing this challenge, LMIC’s Strategic Plan prioritized collaborating with partners and stakeholders to explore the feasibility of options to provide more granular and localized LMI. This edition of LMI Insights, prepared by LMIC and Statistics Canada, identifies and evaluates three broad approaches and lays out the next steps in closing this important gap. Improving information in this regard will help policy makers, educators, career development practitioners and Canadians in general make more informed career, training, and education decisions.
In an effort to lay the groundwork for this Insight, a recent LMIC blog post clarified the four distinct, often confused criteria used to compare labour market information (see Table 1). As we think about how the various approaches stack up against these criteria, it is important to note the inherent trade-offs among them. For instance, more granular LMI might be possible, but only by compromising timeliness. In each case, the overarching limiting factor is the reliability of the information. For example, maximizing localness at the expense of a robust estimation of, say, the unemployment rate is never advisable. Thus, a reasonable degree of reliability must be met when enhancing any of the four criteria below.
Table 1. Four Comparison Criteria of LMI
Localness: | The smallest geographic level |
Granularity: | The number and detail of categories by which data can be grouped (e.g., National Occupational Classification (NOC), age, education level, immigration status, etc.) |
Frequency: | How often the data are available (e.g., monthly, annually) |
Timeliness: | The time lag between the data reference period and data availability |
Three Approaches
Against these four criteria, this edition of LMI Insights examines a number of options to enhance the overall quality of labour market information. In particular, we explore three broad approaches:
- New or extended surveys
- Creating new linkages across existing administrative datasets
- Applying modelling and statistical techniques to generate more accurate estimates
Within each approach, specific options have been identified and expanded upon. The remainder of this article focuses on evaluating each option against localness, granularity, frequency, and timeliness, as well as taking into account costs and statistical rigour. A summary of this evaluation is presented in Table 2.
Table 2. Evaluation of the Current Data Landscape and Three Broad Approaches
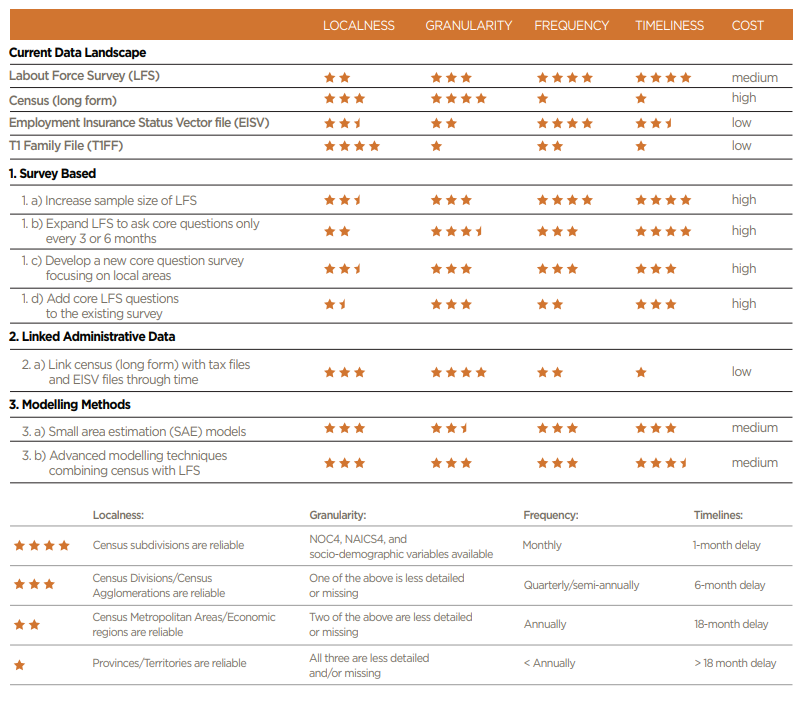
Survey-Based Approach: New or Extended Surveys
Increase sample size of LFS
The first option to consider is increasing the sample size of the Labour Force Survey (LFS). Expanding the survey (currently 54,000 households) has its limitations, however. Given its current structure, it is reasonable that the current breadth of available indicators could be reliably attained for cities with 10,000 to 100,000 residents (i.e., Census Agglomerations [CAs]) by increasing the sample size. This would improve localness compared to current estimates available at the Economic Region (ER) and Census Metropolitan Area (CMA) levels.1 As such, this option improves localness while maintaining granularity and timeliness.
Expand LFS to ask core questions only every three or six months
An alternative option is to pose a subset of the current LFS questions to a larger sample but only on a quarterly or semi-annual basis rather than monthly. This would also make it possible to add new questions on such things as job quality while not compromising the monthly collection and processing of the current LFS. This option provides more granular LMI than the original LFS but is less timely. This option could be designed crosssectionally (samples are different in each cycle) or longitudinally (follow samples for a few cycles).
Develop a new core question survey focusing on local areas
The third survey-based option is to develop a new quarterly or semi-annual survey focusing on smaller geographic areas than is done currently. The questionnaire would use the existing core questions of the LFS in order to maintain comparability between survey results. This option would principally improve localness (although granularity would improve in the cases related to the core questions). The new survey, however, would be collected less frequently (three or six months) compared to monthly.
Add core LFS questions to the existing survey
Finally, we have also considered the feasibility of leveraging an existing survey sample structure by adding a subset of LFS questions. It would be important to use a survey that could improve upon the localness of the LFS without compromising frequency and timeliness (while also not compromising the original survey). However, no suitable survey could be identified that would meet these requirements, particularly in enhancing the localness that already prevails in the LFS estimates.
Putting it all together: The survey-based approach
Among the survey-based options, expanding the size of the LFS offers the most promise — at least in terms of improving upon the established criteria. This option uses the existing survey infrastructure, and the new data — available monthly — would be comparable to all historic LFS data. Altering the scale of the LFS, however, could jeopardize the survey’s robustness and timeliness, as well as increase the response burden. In addition, any structural changes to LFS can only be addressed in the lead up to the next LFS sampling cycle set to begin in 2025. Finally, as with all surveybased options, the cost of expanding the LFS is high, offering less improvement over the current suite of data relative to other approaches discussed here.
Linked Administrative Data Approach
Link census (long form) with tax files and EISV files through time2
Linking various administrative datasets together leverages the highest quality data from each individual source. From largest to smallest sample size, the three data sources offering the most promise for improving labour market information include the following: 1) T1 Family File (T1FF) and T4 files, 2) the long-form Census of Population (Census), and 3) Employment Insurance Status Vector file (EISV).
The T1 Family File and T4 files contain anonymized tax data on all Canadian tax filers (approximately 26 million per year) which Statistics Canada obtains from the Canada Revenue Agency (CRA). These data contain information related to income earned during each calendar year, including employment income, interest, dividends, and rental income. The long form census is a mandatory questionnaire distributed to 25% of Canadian households in 016, roughly 8.7 million people. The long form census gathers granular information on numerous demographics, social and economic indicators such as ethnicity, educational level, and current occupation. Finally, the EI Status Vector file consists of weekly records of EI program participants (an estimated 1 million people per year). In addition to income support for unemployed workers, the EISV includes observations of people using EI in relation to specific life events (e.g., illness, pregnancy, caring for a critically ill family member, etc.).
Although the long-form census is the largest and most detailed survey in Canada (covering 8.7 million individuals in 2016), tax files cover a much larger number of people (approximately 26 million). Therefore, the census sample size is the limiting factor when merging with the T1FF and T4 files (see Figure 1). Then by adding EISV one obtains greater detail about the labour market status of EI users. In order to not limit the linked data to only those users, it is recommended that all non-EI users be kept in the dataset as shown in Figure 1, albeit with less granularity.
Importantly, the linkage between the longform census and the administrative tax and EISV files should be done across time in order to acquire observations between census years.
Figure 1: Overview of linked administrative data option
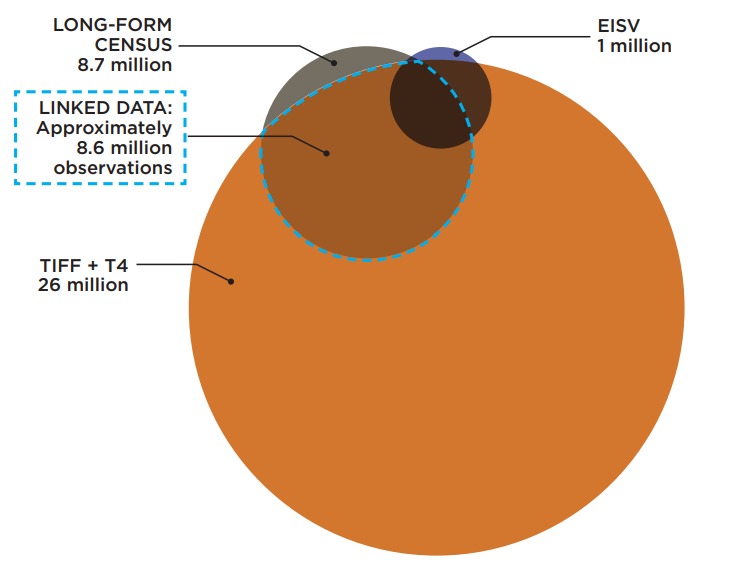
Putting it all together: The linked administrative data approach
Due to the sheer size of administrative data — particularly the census and tax files — the linked administrative data option offers very localized data. Further, because this option leverages existing data, it is rather inexpensive to implement. The challenge of linked datasets is that the four LMI criteria are constrained by the most limited dataset. For example, the number of tax files is so vast that reliable and anonymized data can be produced at the census subdivision or forward sortation area (FSA)3 level. However, the granularity of information is limited to gender and age.
On the other hand, the census offers less localized data, but it is extremely granular: ethnicity, education, occupation, and other characteristics are all observable. Linking these two datasets limits localness to the lowest common denominator among the two (i.e., census divisions and census agglomerations).
In census years, linked census-tax file information provides extremely granular data. For all other years, however, only fixed characteristics (e.g., country of birth, ethnicity) from the census will be applicable to tax record observations. One possible workaround to this dearth of granularity in non-census years is to leverage information from other sources. For example, changeable information such as education level and occupation from the LFS could be linked to tax information in non-census years. The problem is that these other, more frequent data sources are much smaller in size and therefore can only be linked to a limited number of tax file observations. One example of this is shown above in Figure 1 for the case of EISV data being linked to the census and T1FF data.
The major limitation of this approach is its timeliness. Current processing time for data, due to their size and complexity, from the census and administrative tax files is between 12 and 18 months after the reference period. Linking these datasets together would add to this time lag and each additionally linked dataset would further increase processing time.
Modelling Methods Approach
Small area estimation (SAE) models
This option would apply small area estimation (SAE) methods to LFS data in areas for which the number of respondents is too few for reliable labour market information estimates. The technique involves using a complementary or auxiliary data source (with a larger sample and related information) that can be leveraged to improve the localness of the LFS data. The improvement in localness would be a function of the auxiliary data. For example, the LFS contains wage information grouped by occupation. Small area estimates using the LFS as the primary dataset with tax files as the auxiliary dataset offer much more localized information. Importantly, the frequency of SAE results may also be limited by the frequency of the auxiliary dataset.
Advanced modelling techniques combining census with LFS
The final option considered here is a twostep modelling technique that leverages the localness and granularity of the 2016 longform Census with the frequent and timely observations of the LFS. Currently, direct and reliable estimates (e.g., income levels) for small areas cannot be made directly with LFS data, as there are too few observations. Conversely, the long-form census offers very local, granular observations but with a 5-year gap between observations.
To overcome these limitations, a mix of small area estimates, and forecasting techniques could be explored. First, census data would be forecasted to the present (also known as “nowcasting”) by incorporating actual observations taken from up-to-date data such as the LFS, macroeconomic accounts (e.g., provincial GDP), and other data sources. In the second step, the forecasted census variables could then be used as the auxiliary data in an SAE model, thereby enhancing localness and granularity without reducing either frequency or timeliness.
Putting it all together: Modelling methods approach
The options considered under this approach use existing and well-developed modelling techniques to estimate granular LMI for local areas. The SAE modelling is already being implemented by Statistics Canada to estimate unemployment rates in small cities. Using SAE methods alone can be somewhat limiting, however, as the auxiliary input is typically the administrative data and thus comes with the caveats discussed above — namely, it is typically several years out of date and provides little granularity in terms of demographic or labour market status.
The second modelling option leverages the granularity of the census by forecasting it up to the present. The use of census data would allow for much finer breakdowns, including small area estimates of, say, earnings, gender, occupation, and education levels. Such estimates would not be possible with standard administrative datasets. The drawback here is that the approach remains untested, so that evaluation and validation would be required to determine the best forecasting techniques to apply. Further, any modelling approach will require additional validation in order also to reduce uncertainties about the imputed nature of the information, which is a particularly important concern in the research community. In addition to standard econometric time series, emerging machine learning algorithms should also be considered and tested.
Balancing All Criteria: Testing the viability of small-area estimation
As mentioned above, any option within the survey-based approach would be costly and changes to LFS are only possible once the next sampling cycle begins in 2025. For its part, the administrative data approach lacks granular information between census years. Further, linking any administrative data with the census will add to processing time, thereby reducing the timeliness of the data. Given these caveats and comparing them with the limited drawbacks of the modelling approaches discussed above, we believe that small area estimation models, plus some combination of forecasting and SAE methods, provide novel and efficient solutions for generating local, granular data that is both frequent and timely. Such modelling methods are not prohibitively expensive to explore and implement should they prove effective and robust.
Given the advantages and possibilities of the modelling options, LMIC and Statistics Canada are jointly pursuing a research project to explore the feasibility of new small area estimations for several key labour market indicators. The main challenge for SAE models is finding auxiliary data that can enhance the reliability of local-level estimates. Notably, the appropriate auxiliary data source differs according to the estimated variable. For example, Employment Insurance (EI) data have been used to estimate unemployment rates in Census Agglomeration (CA) areas in the LFS. Another possibility is to use provincial and territorial administrative data as an auxiliary data input for the SAE models.
The Way Forward
The Labour Statistics Division at Statistics Canada and LMIC have evaluated a variety of options to provide more local, granular, frequent, and timely LMI. Three broad approaches were identified: survey-based options, linking administrative data, and modelling techniques.
After considering the trade-offs of the three approaches — including potential costs and ramifications to the existing statistical infrastructure — we have begun to work on a joint project to fully assess the possibilities that SAE modelling techniques can offer. The first step entails leveraging administrative data linkages and new forecasting techniques to improve the timeliness of the information generated. In this way, we can leverage the benefits of prevailing sources of labour market information, such as the frequency of the Labour Force Survey and the granularity of the census.
Following this exploratory process, we will engage with our stakeholders to ensure that — based on the criteria set out above — the estimates generated are relevant. To achieve this, the results must adequately address the gap in local, granular, frequent, and timely LMI. When all this preliminary work is done, we will assess the feasibility of scaling this method (should it prove useful) or re-assess the options presented here and chart a path forward accordingly
Acknowledgements
This issue of LMI Insights was prepared jointly by LMIC and Statistics Canada. We would like to thank our federal, provincial, and territorial partners for their comments and suggestions on an earlier draft of this report. In particular, the team would like to acknowledge the valuable feedback and input from Ted McDonald, University of New Brunswick, and Fraser Summerfield, St. Francis Xavier University. For more information about this issue of LMI Insights, please check out our Publications page, contact Behnoush Amery, Senior Economist at behnoush.amery@lmic-cimt.ca, Tony Bonen, Director, Research, Data and Analytics at tony.bonen@lmic-cimt.ca or Josée Bégin, Director, Labour Statistics Division at josee.begin@canada.ca and Vince Dale, Assistant Director, Labour Statistics Division at vincent.dale@canada.ca.
End Notes
- Both CMAs and CAs are urban centres. CMAs are major urban centres with a population of 100,000 or more. Canada currently has 35 CMAs. CAs are smaller urban centres, all of which have between 10,000 and 100,000 inhabitants. There are 114 CAs in Canada. The 76 Economic Regions (ER) in Canada vary widely in area and population. Yukon is the least populous ER with approximately 36,000 people, whereas Toronto contains over 6.2 million inhabitants (2016).
- Strictly speaking the census is not administrative data. It is a mandatory survey. However, given the size and breath of the census, it includes many of the characteristics of large administrative datasets. We therefore include it while discussing linking administrative datasets.
- Forward sortation areas (FSAs) are the first three digits of a postal code.