Table of Contents
Key Issues and Findings
- The Education and Labour Market Longitudinal Platform (ELMLP) is a unique data environment that will help us better understand the transitions of Canadians from education and training programs into the labour market.
- Currently, the ELMLP provides access to three anonymized administrative datasets linked through time: 1) the Post-secondary Student Information System (PSIS); 2) the Registered Apprenticeship Information System (RAIS); and, 3) T1 Family File tax records (T1FF).
- With its ability to bring together longitudinal data, ELMLP offers an extraordinary opportunity to analyze how individuals fare in terms of income level and growth following their participation in a university, college, or apprenticeship program.
- Using administrative data, ELMLP provides comprehensive coverage of enrollees in public postsecondary education and apprenticeship training programs in Canada - with considerable additional potential linkages possible. This will enable researchers to uncover new and specific factors of successful transitions to the labour market.
- The existing datasets in ELMLP nevertheless come with certain limitations, such as lack of data on individual jobs (e.g., no occupational data) and limited "work quality" indicators (e.g., no hours worked, benefits, or workplace culture information). Further, the pan-Canadian set of PSIS is only available for the 2009/10 to 2015/16 academic years, and RAIS for the 2008 to 2016 calendar years, limiting - at least for now - the duration of the longitudinal analysis.
Introduction
In a recent blog, LMI and Microdata Linkages, we explored the potential power of linking various administrative datasets. To that end, the 2018 Federal Budget included significant investments to establish the Education and Labour Market Longitudinal Platform (ELMLP). ELMLP is a linkage platform in which separate data files can be merged by researchers with an anonymized master key. In November 2018, the platform became accessible in Statistics Canada’s Research Data Centres (RDC). Researchers are now able to access data from this platform by submitting a research proposal, passing an RCMP background check, and becoming a "deemed employee" of Statistics Canada. Once approved, researchers can access the platform only in the highly secured RDC environments located in select universities across the country and at Statistics Canada’s headquarters in Ottawa.
The platform currently brings together three core administrative datasets that researchers and data analysts can link together and through time. These are:
- PSIS: The Post-secondary Student Information System, which includes records of college and university students’ programs, credentials, and fields of study
- RAIS: The Registered Apprenticeship Information System, which includes records on registered apprentices and trade qualifiers in apprenticeship programs, by designated trade.
- T1FF: The T1 Family Files, which are tax files that include information on labour and non-labour income, industry of work (3-digit NAICS, the North American Industry Classification System), as well as indicators such as Employment Insurance and social assistance support
Each dataset also contains core sociodemographic variables such as age and gender and, in PSIS, some information about individual student status (e.g., Canadian citizen or student visa in PSIS; see Table 1).
The ELMLP environment will enable LMIC and other researchers to uncover new insights regarding the transitions of students and registered apprentices into the labour market. Greater discussion of the possibilities and limitations of such analyses are discussed below, but first we present some details about the information available in each of the three data sources that comprise the platform.
A Glimpse at the Data
The three data sources comprising ELMLP bring together a set of complementary information related to education, training, and earnings. A list of key variables available in each dataset is provided in Table 1. Note that the list provided is by no means exhaustive, as the platform contains a wide array of detailed information.
The Post-secondary Student Information System (PSIS) data include records from over 300 public colleges and universities in all provinces and territories covering the academic years 2009/10 through 2015/16. Further, for institutions in the Maritimes, PSIS data are available from 2005/06 to 2015/16. PSIS data include information on student program status (enrolled, graduated, etc.), international student status, and field of study in each academic year.
The Registered Apprenticeship Information System (RAIS) contains information on workers enrolled in trades apprenticeships, including in-class and on-the-job training, as well as information on trade qualifiers. RAIS includes information on Red Seal and non-Red Seal apprenticeships, and indicates whether the apprenticeship training is compulsory or voluntary. Red Seal apprenticeships usually include between 2 and 5 years of work integrated learning (WIL) that is standardized across provinces and territories. The RAIS data include information on apprentice status (continuing, completed, etc.), trade of apprenticeship, and the broad occupational category (National Occupational Classification (NOC), 2016), associated with the trade. RAIS data are available from calendar year 2008 to 2016.
The T1 Family File (T1FF) of tax records contain information on individual earnings by category, including employment income and social support income from Employment Insurance (EI) and social
assistance programs.
In the initial release of ELMLP, T1FF data will include tax records from 2004 to 2015 for PSIS (in March 2019, T1FF data for 2016 will be added) and up to 2016 for RAIS. When an individual first appears in PSIS or RAIS, their T1FF data become available from that tax year through to 2015 or 2016, respectively. Further, if T1FF data are available for an individual in years prior to their first appearance in PSIS or RAIS, the platform allows linkages to these earlier observations as well.
Table 1. Summary of types of information and variables within ELMLP
PSIS | RAIS | T1FF | |
---|---|---|---|
Time and status |
|
|
|
Primary variables |
|
|
|
Socio-demographic |
|
|
|
Age and gender |
Opportunities to Assess Outcomes
To leverage the platform’s longitudinal structure, researchers can pursue two broad approaches. The first is "years since completion" or, more generally, years since an educational or training program was exited (through graduation, completion, or any other reason). An alternative approach is to conduct a "year-specific analyses" that focuses on labour market outcomes, in a particular tax year, across different cohorts who had previously participated in post-secondary education and/ or training programs. In both approaches, individuals may be grouped across demographic characteristics, program types, or fields/trades of study.
Using both approaches, we will be able to track and assess returns to, and premiums on, participating in education and training programs;for example, the relative earnings across different fields of study or different types of degrees. Similarly, it will be possible to compare earnings levels for those who graduated versus those who did not complete their program. Finally, it will be of interest to many researchers to quantify the earnings trajectories of various underrepresented groups (e.g., women, immigrants, etc.) after controlling for factors such as field of study and program completion.
Beyond income level itself, labour market outcomes analyses could focus on earnings volatility. Here a year-specific analysis is likely more suitable as earnings volatilities are heavily influenced by current macroeconomic conditions (e.g., periods of low growth versus strong economic times). Earnings volatility could be proxied in a number of ways, including annual changes in income, accessing EI or social assistance, or the number of T4s reported in each year.
Doubtless, there are numerous other possibilities for analysis that we have yet to consider. While an exhaustive list is beyond the scope of this edition of Insights, other fruitful avenues of investigation include the following:
- The distribution of former students and apprentices who experience periods of low earnings
- The social mobility of individuals relative to their family income level (available for PSISlinked T1FF data)
We hope researchers from across the country delve deeply into the platform and share their findings broadly. Our collective actions - both independent and in collaboration - will yield many new labour market insights to inform comprehensive data-driven policy development. Indeed, preliminary results have already shed light on the information-generating power of the platform (Box 1).
Box 1: Initial ELMLP Findings
On 4 December 2018 Statistics Canada released the first public findings from the data linkages in ELMLP. They report that from 2010 to 2014 over 900,000 students under the age of 35 graduated from a postsecondary institution and joined the labour market. The median employment income two years after graduation was $43,600 for those with undergraduate degrees (53% of total observations) and $39,100 for college-level diploma holders (14% of total observations). A second key finding is the level of the gender income gap among both college and university degree holders. Five years after graduation, women with undergraduate degrees earned 21.1% (or, $13,300/year) less than their male counterparts, and women with a college diploma earned 29.8% (or, $16,200/year) less than men with the same educational attainment.
Limits and Caveats
Although ELMLP provides numerous opportunities to assess labour market outcomes of students and registered apprentices, the platform comes with several important limitations that should be borne in mind.
Occupational Information
The employment information in ELMLP is limited. While earnings data are robust, the T1FF does not contain any information on occupation. The T1FF data do provide industry information, which is coded as a 3-digit NAICS. While it provides some indication of training/job match, the NAICS3 alone is not sufficient to determine if an individual works in a field related to their education or training program. For example, from PSIS we can observe people holding degrees in computer science (CIP: 11.0701) who, based on T1FF, now work in the commercial banking sector (NAICS3: 522). Without a National Occupational Category (NOC) code, we cannot know if these people are working in the IT department of banks, as analysts in another department, or as senior managers. Future data to be integrated into the platform, such as the Census or the National raduate Survey (NGS), will help close this information gap.
Work Quality and Quantity
In addition to the dearth of occupational information, the platform contains no information on the quality or quantity of work. There are no data, for example, on the hours worked, flexibility of schedule, or even volatility of earnings within a tax year. Indeed, other important factors such as work-life balance or the number of jobs held simultaneously are also absent from the ELMLP datasets. In this respect, the addition of the Labour Force Survey (LFS) to the platform could help and, at least for a subset of the population, improve the availability of work quality and quantity data. The addition of the Census or NGS to the platform could also be used to determine if a graduate is working full-time or part-time (and if so, why), if the job permanent or not, and other related job aspects.
Worker Skills
Generally, administrative data provide little information on skills, which must be self-reported or measured through testing. One work around would be to use the "skill level" associated with each 4-digit NOC code, were they to become available via the Census or LFS in future updates to ELMLP. However, as pointed out in LMI Insight No. 3, direct measures for a narrow set of skills are increasingly possible through online testing, but this remains a persistent gap in Canada’s system of labour market information.
Earnings Comparisons Group
While income levels and changes are the central element of available analyses in the platform, an earnings comparison group would be developed. Ideally, the earnings of participants in education and training programs should be compared to individuals who have not participated in such programs. Unfortunately, the platform’s T1FF data are available only for those in the PSIS/RAIS universe. At a minimum, one could compare the earnings of students who started studies but did not complete them.
Even if researchers had direct access to the T1FF information of such comparison groups, it is not clear how to align the cohorts in ELMLP with those outside of it. Age, gender, and sector would be the obvious characteristics by which to align the comparison groups (e.g., 25-year-old women working in the mining sector inside and outside of the PSIS/RAIS universe). Yet, the comparison here would not be perfect, as earnings are typically measured based on the years since entering the workforce, which is very different for former students, apprentices, and those who started working directly out of high school.
Time Coverage
A related problem to the earnings-comparison group issue is that analyses based on "years since graduation" lump together individuals across very different macroeconomic circumstances. Consider the PSIS data as displayed in Table 2. For all jurisdictions, ELMLP has one cohort with five full years of post-education earnings information (2009/10 graduates); all others have four or fewer full years of earnings information. Analyzing earnings in t+1 years since graduation, would include graduates from 2009/10 through 2015/16. That is, earnings at t+1 counts
individuals graduating into the post-financial crisis economy as well as those graduating into 2015’s tightening labour market. Controlling for these macroeconomic conditions is an important but not entirely straightforward procedure.
Cost of Education
The last but perhaps most important caveat to earnings premium analyses is that researchers should account, to the extent possible, for the direct (e.g., tuition, fees) and indirect costs (e.g., foregone earnings) associated with their education and training program. Educational costs are not included in the platform, and the opportunity cost of earnings is, of course, unobservable. Accommodation costs are also excluded from these data sources for students not living with parents while attending school. Wellreasoned assumptions will have to be introduced by researchers to adjust adequately and accurately for such costs.
Table 2. PSIS-T1FF time table between years of graduation (PSIS) and years of employment income (T1FF) to show the potential years of analysis after graduation for each cohort
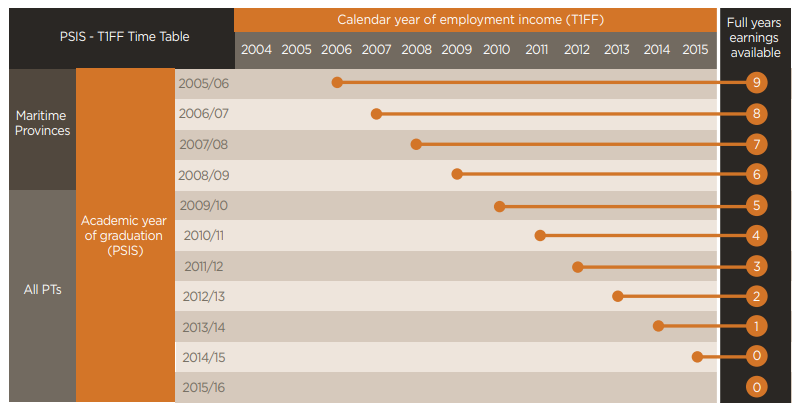
Future Linkages
Some of the caveats and limitations noted above could be ameliorated with the inclusion of additional datasets. Certain datasets are already being considered for integration into the platform1:
- Canadian Student Loans Program data (to be made available in RDC in February 2019)
- Canadian Education Saving Program data (expected to be available in RDC in fall 2020)
- Canada Apprentice Loan and Apprenticeship Grant Program data
- Employment Insurance Claimants, Record of Employment, and Employment Insurance Status Vector data
- Select information from the Census and National Household Survey
Although these supplementary datasets also have their own limitations and caveats, integrating them into the platform would address the following two data gaps: 1) the cost of educational and training programs; and 2) occupation-specific information. The former would be covered by administrative data on student loans, which is an important financial consideration to bring to any analysis of net earnings and returns to education. The latter data gap would be sourced from the 2016 Census and 2011 National Household Survey (NHS). However, these two surveys introduce additional limitations. First, the Census and NHS cover 25% and 33% of households, respectively. Second, the occupational information in these datasets is available only every five years.
An alternative data source that could be integrated into ELMLP is, as mentioned above, the Labour Force Survey (LFS). While its coverage of the population is far smaller than the Census or NHS, the LFS would offer more up-to-date data on occupational categories. Notably, integration of the LFS would also enable some measures of hours worked. Similarly, Employment Insurance (EI) data would add occupational details for those who have used EI, while also providing information about the duration of people’s unemployment spells.
The Way Forward
The Education and Labour Market Longitudinal Platform (ELMLP) is an important and rich initiative that will provide empirical evidence and support research and insights - from a variety of perspectives - for numerous stakeholders and individuals interested in the transitions of students and registered apprentices into the labour market. To that end, LMIC plans to launch a series of in-depth analyses of labour market outcomes in partnership with recognized experts from the Education Policy Research Initiative (EPRI) and Statistics Canada.
It will be critical, however, to contextualize any initial insights drawn from the platform with appropriate comparison groups and clearly spelled out caveats of the analysis. Such contextualization is imperative for any of the results to be meaningful to stakeholders, policy makers, students, and the broader Canadian public. For this reason, we look forward to new datasets being integrated into the platform to address prevailing information gaps and expand the breadth of research possibilities.
Along with the full research community, LMIC is excited to delve into the platform, share our insights (with the appropriate emphasis on limitations) and to tailor that information in a way that addresses the diversity of users’ needs.
Acknowledgements
This issue of LMI Insights was prepared by Behnoush Amery of LMIC. We would like to thank our National Stakeholder Advisory Panel, Labour Market Information Experts Panel and Statistics Canada for their comments and suggestions. In particular, the team would like to acknowledge the valuable feedback and input of Sylvie Gauthier, Christine Hinchley, Tamara Knighton, André Lebel (Statistics Canada), Ross Finnie, Michael Dubois, Masashi Miyairi (Education Policy Research Initiative) and Arthur Sweetman (McMaster University).
For more information about this issue of LMI Insights or other LMIC activities please check out our Publications page, contact Behnoush Amery, Senior Economist at behnoush.amery@lmic-cimt.ca or Tony Bonen, Director, Research and Analytics at tony.bonen@lmic-cimt.ca.
End Notes
1Based on the Education and Labour Market Longitudinal Platform’s governance documents which are not yet available to the public.