April 2022
Developing Indicators for Skill Demand
Three approaches to developing indicators for skill demand using online job posting data.
Key Findings
- However, skill proxies are not appropriate for assessing skill demand because they are not based on actual skills; and
- occupational profiles, although a source of specific skills data, require additional data for insight into skill demand.
- Raw count ordering;
- Revealed comparative advantage (RCA); and
- Term frequency–inverse document frequency (TF–IDF).
Table of contents
Introduction
As Canada recovers from the impacts of the global pandemic, adjustment to the post-COVID world of work is increasing the need for labour market information (LMI) that can help Canadians and businesses recover rapidly.
This includes high-quality metrics that indicate which skills are in demand.
With accurate and accessible skills information, Canadians can develop the competencies needed to find jobs that are right for them and to succeed in the world of work — ultimately improving their quality of life and supporting Canada’s continued economic success.
Measures for skill demand, however, are almost non-existent. This is partly due to limitations in the availability and types of existing skills information.
Building on our previous research on skills shortages, linking skills to occupations and the representativeness of online job posting data, this report outlines the most common sources for obtaining information on the skills requirements of jobs. It then explores how this information could be leveraged to create measures, or indicators, of skill demand.
Three approaches in particular merit further study: raw count ordering, revealed comparative advantage (RCA) and term frequency–inverse document frequency (TF–IDF).
Not All Skill Indicators Are Equal: Skills Proxies and Occupational Profiles
The call for skills information is not new, and while some indicators exist, they are not always based on direct skills information.
When it comes to developing reliable skills indicators, the source of information is critical. The most common sources of skills information are:
- skill proxies, such as level of education or field of study; and
- skills information linked to occupational profiles.1
The latter source includes data sets like the US Occupational Information Network (O*NET), the European Skills/Competences, Qualifications and Occupations (ESCO) and Employment and Social Development Canada’s forthcoming Occupational and Skills Information System (OaSIS).
Skill proxies do not measure skills directly and, even more problematically, proxies tend to limit “skills” to a single dimension.
Simply identifying the level of education required for an occupation, for example, is insufficient. Individuals, educators and employers want information about which skills they should invest in, develop and seek out. In fact, in the past few years, there has been a growing trend away from skill proxies.
Occupational profiles, on the other hand, are a better source because they identify specific skills. O*NET, for example, provides a taxonomy of 35 skills (as well as other job descriptors) rated for importance and complexity (i.e., level) for over 900 occupations — based on surveys of workers.
Occupation-linked information systems — like O*NET, ESCO and OaSIS — are designed to support career planning by providing descriptions of various job characteristics, including skill requirements, job tasks and work activities, to name a few. Figure 1 lists the five skills with the highest importance and level (i.e., complexity) ratings for registered nurses and psychiatric nurses from O*NET, illustrating the type of skills information that can be derived from an occupational profile.
Figure 1: Top Skills for Registered Nurses and Psychiatric Nurses (NOC 3012)
Active Listening and Social Perceptiveness
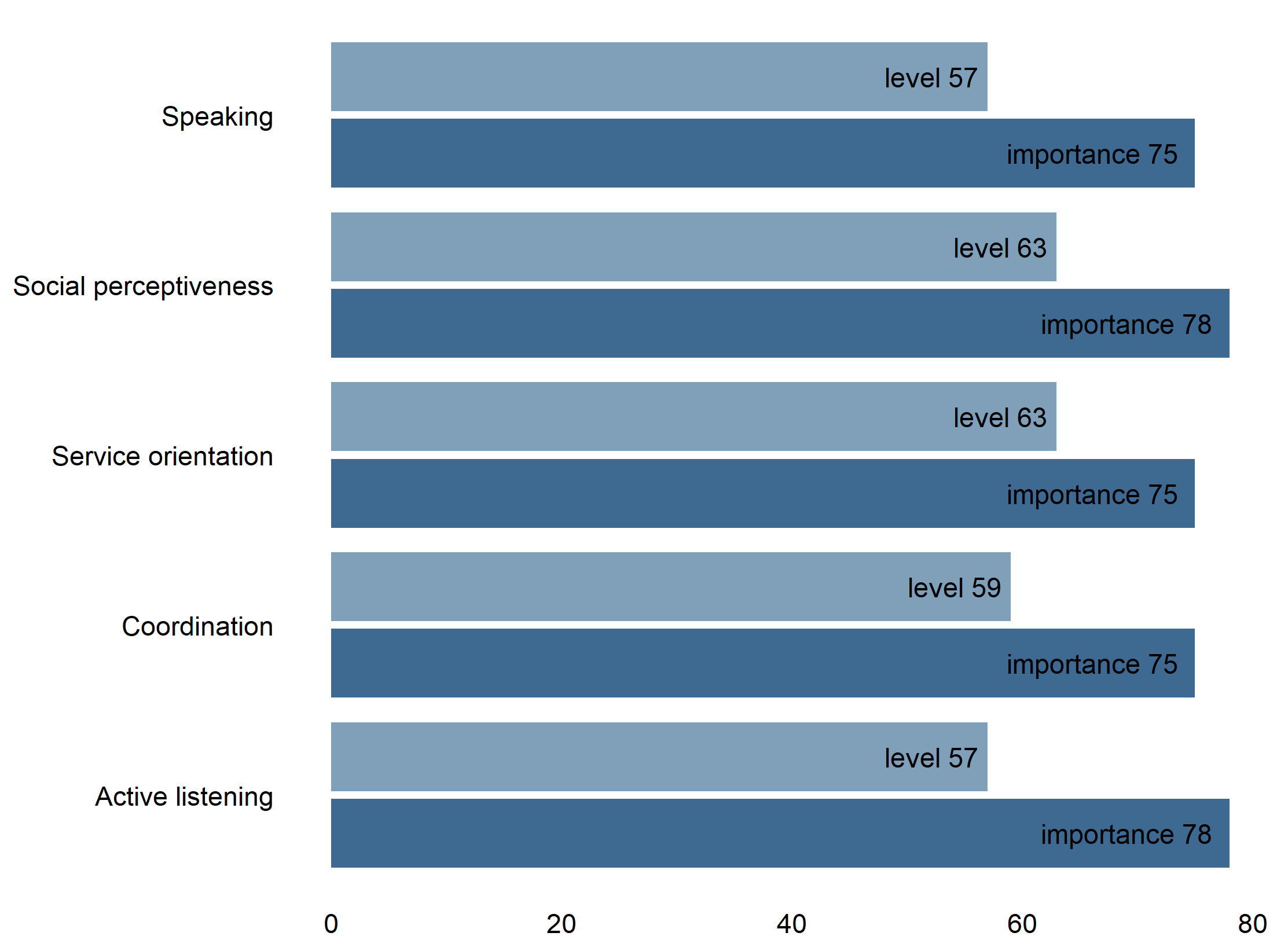
Despite the obvious advantages of occupational profiles over skill proxies, some limitations do exist.
First, the skills are static, coming from a pre-defined taxonomy and rated for the occupation.
Second, importance is not equivalent to demand. The fact that “active listening” is considered one of the most important skills for nurses does not necessarily mean it is in demand. Employers must be seeking out skills to imply demand.
In addition, ratings in occupational profiles are infrequently updated (every five years in O*NET) and lack historical, regional or industry-specific dimensions — limiting the analysis of skills.
Consequently, data derived from occupational profiles require additional sources of data (e.g., employment, wages) to develop insights, including the demand for skills.
To measure the demand for “social perceptiveness,” for example, one must estimate the aggregate demand for which social perceptiveness is of importance to an occupation. Occupations with ratings of 75 or higher might be chosen.
Using another data source, such as the Labour Force Survey, Census or Job Vacancy Wage Survey, one could then examine the employment levels of all relevant occupations to determine the trend over time. However, this approach measures employment growth among a group of occupations, identified by a shared skills trait (social perceptiveness >= 75), rather than the growth of the skill itself.
This is not to say that occupational profiles and their associated skills information are not useful. Rather, it is important to understand that, for certain insights, data derived from occupational profiles requires additional information, such as employment, vacancies and average wages. One notable example of a skills indicator developed from occupational profile data is the OECD’s Skills for Jobs indicator (see Box 1).
Box 1: OECD's Skills for Jobs Indicator
To provide more detailed information on labour market skill demand (and supply), the Organisation for Economic Co-operation and Development (OECD) constructed a database of skill needs and skill mismatch indicators.
The skill needs indicator identifies skills shortages and surpluses based on five labour market signals — the growth of wages, number of jobs and hours worked, as well as the rates of unemployment and under-qualification — that can be used to predict a shortage, surplus, or balance of labour for a given occupation. The indicators are calculated at the 2-digit International Standard Classification of Occupations (ISCO) occupational level (containing 33 occupation groups).
Next, to leverage the skill importance ratings from O*NET, a public concordance to map from ISCO to O*NET is used. Thus, for an occupation determined to be in shortage, those skills rated most important are interpreted as being “in-demand.” If, for example, there is a demand for nurses, one might conclude that there is a demand for their skills, with the highest rated skills being most in demand (see Figure 1).
Lastly, this information is aggregated at the country level — using employment shares to weight the skill demand by occupation. This results in an indicator that shows the direction (surplus or shortage) and magnitude of the need for skills in each country.
Online job postings as a source for skills data
Developing indicators that are more reliable, accessible and directly linked to skill demand is a challenge because we do not directly observe a market for skills. Rather we observe job markets in which skills are bundled to represent an occupation.
For this reason, online job postings have gained much attention since they represent a direct source of skill demand data.
Beyond skills, online job postings provide insights into a wide range of work requirements such as knowledge domains, tools and technology, and workplace conditions. This information is gathered at the job posting level, which may also be linked to an occupation, city, industry, offered wage and other features, enabling further analysis.
LMIC’s Canadian Job Trends Dashboard, for example, includes skills aggregated by occupation. In addition, job posting data are updated weekly, are linked to local labour markets and are delivered within a few days at a fraction of the cost of traditional surveys.
However, important caveats remain:
- First, online job postings reflect the language of employers and HR departments, meaning any “assumed” or “implicitly” required skill will not be listed in a job posting and hence not measured.
- Second, the data are noisy: Job postings are cheap for employers to post and may reflect multiple openings per posting or no openings at all if the employer is simply “seeing what’s out there.”
- Third, the skills information derived reflects only the online job postings market — meaning the data may under- or overrepresent industries, occupations, regions and firm sizes when compared to actual vacancies. Therefore, great care is needed in linking skills derived from online job posting data to other labour market indicators, such as employment conditions, hours worked or average wages, as well as in the conceptual and technological methods used to identify and categorize skills.
More information on the advantages and limitations of using online job posting data can be found in recent LMIC publications and the background documentation accompanying our Canadian Job Trends Dashboard.
With these caveats in mind, we examine three approaches for developing indicators of skill demand from online job posting data: 1) raw count of skills (or the frequency of skills per dimension); 2) revealed comparative advantage (RCA); and 3) term frequency–inverse document frequency (TF–IDF). Table 1 outlines these three methods.
Table 1: Approaches to Identifying In-Demand Skills
Approach | Description | Key benefits | Key drawbacks |
Raw Count Ordering | Ranking skill demand by the frequency in which they appear in job postings | Easy to compute and understand | May overrepresent frequently listed skills |
Revealed Comparative Advantage (RCA) | Ranking skill demand relative to other skills | More informative regarding how relevant or unique a skill is | Could magnify problems with imperfect algorithms and/or poorly written job ads |
Term Frequency–Inverse Document Frequency (TF–IDF) | Ranking skill demand relative to other skills | More informative regarding how relevant or unique a skill is | Results can be overly sensitive since the frequency of terms in other documents is not considered |
Using Online job postings, Approach 1: Raw Counting Ordering of Data from online job postings
Raw count ordering simply ranks skills by the frequency with which they appear in online job postings (see Table 2). This frequency — a common indicator of skill demand — can be observed by occupation, job title, industry, region and/or any other feature linked to job postings. Most often raw counts are converted into frequencies within a category (e.g., “customer service” appears in 26% of postings for payroll clerks in Victoria, B.C.).
The raw count indicator for in-demand skills is simple to calculate and to understand. Skills information in job postings is treated as a direct measure of demand.
For example, if skill X appears twice as often as skill Y, then the demand for X is twice that for Y. However, online job postings do not reflect the job market per se. Instead, they reflect the language used in job postings, including implicit biases and generalized recruitment language.
Consequently, frequently listed skills, like communication or teamwork, rank highly because of how often they appear in postings.
Are these skills the most “in demand”? On the surface, yes: nearly every job requires communication and teamwork. However, these skills are not necessarily in demand relative to supply or relative to a particular occupation or industry.
In many cases, this kind of relative demand is useful in shedding light on the skills that set one job apart from another, helping to identify a skill’s marginal value in a sought-after occupation or industry.
The approaches discussed here build on the raw count information but transform these counts to produce measures of relative demand at the skill or group level (e.g., occupation).
Using Online job postings, Approach 2: Revealed comparative advantage (RCA)
Recent research has drawn on Revealed Comparative Advantage (RCA) as a measure of relative skill demand. RCA emerged in other areas of economics, such as trade and industrial organization but has more recently been applied to online job posting data.
In the skills setting, RCA is the ratio between (a) the relative frequency a skill appears within a job posting and (b) the frequency that skill appears across all job ads relative to all skills. Thus an RCA value can be calculated for every job posting–skill pair, with values greater than 1 indicating a higher-than-average skill frequency for that job ad (i.e., higher than average skill demand; see Table 2).
For example, if “critical thinking” appears in a job ad at ABC Inc. with only one other skill (e.g., “teamwork”) then the numerator of the RCA ratio is ½. To get the RCA denominator, let’s say that “critical thinking” appears 10 times across 20 job ads (including the ABC Inc. ad) and 30 unique skills are mentioned. The RCA denominator is 10/30, making the RCA value for “critical thinking” for our single job ad at ABC Inc. [(1/2)/(10/30)] = 1.5.
The RCA value for “critical thinking” in this job ad at ABC Inc. is greater than 1 because this one skill represented a higher share of those demanded in the posting than among all unique skills in all postings.
Typically, skills are “in-demand” if their RCA value in a job ad is greater than or equal to 1, and “not in-demand” otherwise. Accordingly, higher values imply higher relative demand as well.
RCA values can also be aggregated to occupations, industries or regions. A potential drawback, however, is that RCA can magnify problems with imperfect algorithms and/or poorly written job ads.
Job ads with only a small number of associated skills (i.e., two or three) will have large values in the RCA numerator and therefore be more likely to have skills deemed “in-demand.” But in fact, the job ad might merely be short or the algorithm for codifying the natural language unable to do so well in this case. In aggregate, this drawback is unlikely to be severe and will lessen as algorithms for gathering job posting data continue to improve.
Using Online job postings, Approach 3: Term Frequency-Inverse document frequency (TF-IDF)
Another approach to measuring relative skill demand is the calculation of term frequency–inverse document frequency (TF–IDF).
TF–IDF is similar to RCA in that it is essentially a simple ratio, where the numerator is the raw count of skills (the term frequency) within a document (a job ad or group of job ads); the denominator is the likelihood that the skill appears in other documents (e.g., job ads). In practice, the TF is multiplied by the inverse document frequency (the IDF) after a log transformation. With online job postings, it is common to treat the occupation as the “document,” but any common feature could be used.
TF–IDF comes from the analysis of natural language texts as a way to identify the most relevant terms (e.g., words or skills) in a document (e.g., books or occupations) compared to a collection of documents.
The most common words in any one book are things like “the,” “she,” “a,” “at,” etc. The same phenomenon occurs with job postings in which “communication” and “teamwork” tend to be the most common attributes listed.
The IDF component acts as a weight on the raw count frequency of each skill. By decreasing the weight for skills that appear often across many postings, the common skills are given a lower value (i.e., lower relative demand) in comparison to others. However, that is not to say that low-ranked skills are less important, simply that they are not unique to the occupation.
Comparing Approaches
With all three approaches, the skills in the online job postings receive a ranking or score that represents the demand for the skill. Unlike raw count or relative frequencies, which have a clear interpretation, RCA and TF–IDF values simply indicate a higher or lower relative demand. However, both TF–IDF and RCA values can also be leveraged quite easily to identify similar skills across occupations — a benefit that separates them from other approaches.
Table 2 reveals skill rankings and scores for registered nurses and psychiatric nurses from raw count ordering, TF–IDF and RCA. Top skills are similar for nurses using raw count frequency and TF–IDF, while RCA offers different results in part due to the methodology’s inclusion of relative skill frequency from online job postings.
Table 2: Top skills for registered nurses and psychiatric nurses (NOC 3012)
Communication, System Monitoring and Critical Thinking
Skills | Frequency* | Skills | RCA | Skills | TF-IDF | ||
Communication | 53% | System Monitoring | 974 | Critical Thinking | 11039 | ||
Leadership | 42% | Advocacy | 920 | Planning | 9643 | ||
Problem Solving | 37% | Reporting | 904 | Problem solving | 8841 | ||
Critical thinking | 34% | Facility management | 611 | Leadership | 8581 | ||
Planning | 33% | Organizational development | 550 | Communication | 8092 |
Next Steps in Developing Indicators of Skill Demand
With accurate and accessible skills information, Canadians can ensure that they develop the competencies needed to succeed in the labour market.
Developing indicators of skill demand is one advancement that can help improve the process. Online job postings offer a promising data source with flexibility in the approaches used to develop these indicators.
LMIC is working with the Future Skills Centre to deliver LMI tools and data — including in-demand skills — to career service professionals. As part of this work, we are building a cloud-based data repository in Google Cloud Storage (GCP) for a wide variety of LMI data. This includes job posting information and new skill indicators such as those discussed in this report. We will test the relevancy of these approaches with our stakeholders going forward.
As we work with partners across the country and beyond, we will continue to develop new skill indicators — including metrics for emerging skills — that will be added to the LMIC Data Hub.
Acknowledgements
This LMI Insight Report was prepared by Michael Willcox of LMIC. We would like to thank Strac Ivanov (Vicinity Jobs), Ana Ferrer (University of Waterloo), Matthias Oschinski (Belongnomics), Jacob Loree (Finance Canada), Marc Frenette (Statistics Canada), Glenda Quinitini and Luca Marcolin (OECD), Ron Samson (Magnet), Marc Gendron (Employment and Social Development Canada), Naomi Pope, Amy Wongkanlayanush and Ermias Afeworki (B.C. Ministry of Advanced Education and Skills Training) for their feedback and constructive comments.
For more information about this report, please contact Michael Willcox, economist, at michael.willcox@lmic-cimt.ca, or Anthony Mantione, director of research (acting), at anthony.mantione@lmic-cimt.ca.
Endnotes
1 Employer surveys are another potential source of skills information but are not currently in use in Canada.