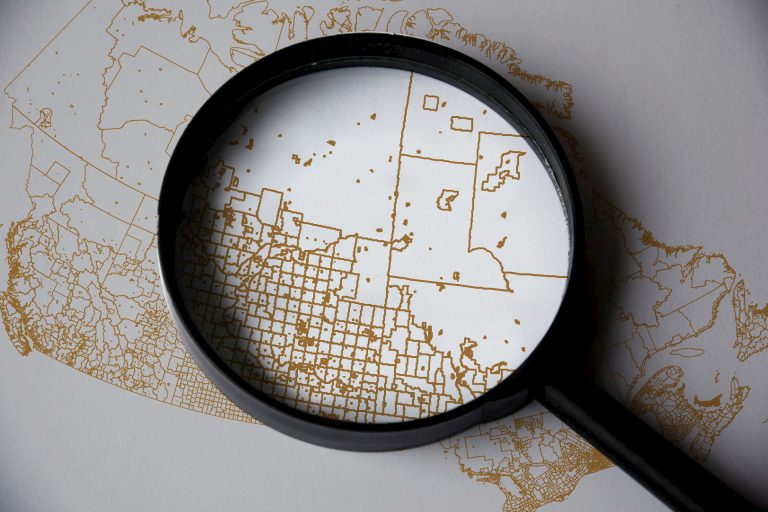
Local, Granular data for Beginners
On my first day at LMIC, I was warmly welcomed by the Research and Analytics team. My new colleagues were excited to tell me about their ongoing data collection and analytics projects — but they did so using strange terms like POR, FOW, ELMLP, and LG. Although I was a little overwhelmed by the number of acronyms, one stood out: LG. After some hesitation, I finally built up the courage to ask my colleagues what LG meant in the context of labour market information (LMI). They explained that it refers to “local, granular” — one of the top priorities when it comes to generating LMI for Canadians.
I had thought the two terms were synonyms but, as my new team explained, at LMIC, local and granular actually refer to distinct concepts:
- Localness is the smallest geographic area for which reliable labour market information is available.
- Granularity refers to the categories by which labour market information can be grouped across individuals, and can be thought of as having two components
1. Labour market categories such as labour market status (employed or unemployed), or occupational groups. This determines the availability of variables such as the unemployment rate and occupation-specific average wages
2. Sociodemographic categories such as gender, age, immigration status, among many others. This type of granularity specifies which labour market variables are reliably available for specific sub-populations in the labour market (e.g., youth unemployment).
Once explained, the LG categories made sense. But I soon learned of two other categories that LMIC uses to determine the quality of data, namely the frequency and timeliness of data. Frequency is how often the data are available, whereas timeliness refers to the delay between the reference period of the data and when it becomes available (e.g., 2015 taxes are available as anonymous data points in 2017, meaning a two-year delay). The four characteristics used to evaluate data are listed in Table 1 below.
Table1: Four Comparison Criteria of LMI
What is Available?
The Labour Force Survey (LFS) is one of the most popular and widely used sources of labour market information in Canada. Produced monthly, it provides some of the most reliable, up-to-date information on the labour market, including the unemployment rate, educational attainment, and income from work.
Like any other survey, the LFS has some limitations. Although the LFS surveys approximately 60,000 households across the country each month, the sample is not big enough for smaller groups or regions. For example, we can estimate labour market variables for the Greater Toronto Area by gender and age, but not for a smaller area within Toronto, such as Scarborough. This means the LFS may not provide the details needed by local policymakers, career practitioners, and other stakeholders who focus on very specific (read: local, granular) contexts. For them, we had to explore alternative data sources.
A Glimpse of What is Possible
There are more than a few relevant large datasets on labour market information in Canada (see my colleague Behnoush’s blog). We have identified the T1 Family File (T1FF) of income taxes, the 2016 Census of the population, and, potentially, the Employment Insurance files as datasets that could provide very local, granular labour market information (LMI). The content of these datasets, as well as the LFS, are summarized in the table below.
The big difference between the LFS and these three datasets is size — and in the world of labour market information that matters. With a bigger dataset, you can group the data in finer ways without sacrificing the reliability of the estimated value. Of course, refinements are possible only if the granular grouping variables are present; for example, you can’t observe the unemployment rate of immigrants if this group isn’t identified in the dataset. Since the Census contains such a wealth of sociodemographic variables, linking it with tax and EI data through time could provide an enormous opportunity to produce local and granular LMI.
Linking Census and administrative datasets together rightly raises the issue of data security and privacy. For these individual datasets and their potential linkages, LMIC — as with all researchers — is subject to strict privacy and data protection protocols that are overseen by Statistics Canada at every step of the process. While local, granular LMI is essential for good policy making, it need not risk the privacy of Canadians.
Going Forward
The opportunity for more local, granular LMI from the Census and administrative datasets is clear, but these sources are much less frequently updated than the LFS. For current snapshots of Canada’s labour markets, the LFS will continue to be the most up-to-date source. For the most detailed information on Canada’s labour markets, large dataset linkages will be the way forward. Administrative and Census data linkages could greatly enhance the localness and granularity of labour market information available. I along with the rest of LMIC is excited to start pursing such data linkages in partnership with Statistics Canada.
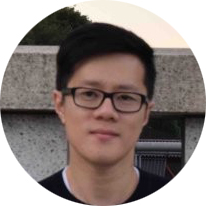
Young Jung is an Economist with LMIC. He is currently providing analytical support on a wide range of labour market issues to help understand and promote labour market development.