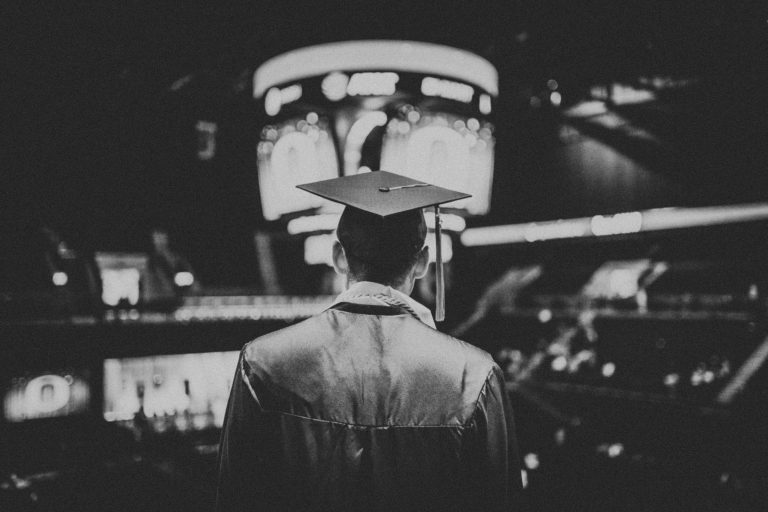
Earnings Comparison Groups
Researchers across the country — myself included — eagerly anticipated the release of the Education and Labour Market Longitudinal Platform (ELMLP) in November last year. By linking postsecondary education (PSE) and apprenticeship training records to individual tax returns, this platform provides a major step forward in addressing what some have called a “data deficit” in Canada.
A recent LMI Insight article by Behnoush Amery offers details about the possibilities and limitations of ELMLP. A key policy question relates to estimating the financial returns from education and how these have changed over time. Dr. Amery notes that the platform lacks a comparison group to estimate the earnings of workers who did not participate in a college, university, or apprenticeship training program. Such individuals constitute a large segment of the workforce, and their earnings provide an important baseline against which to assess the returns to education and training.
My team at the New Brunswick Institute for Research, Data and Training (NB-IRDT) at the University of New Brunswick has been working with LMIC to find solutions to this data limitation. As one would expect, the quality of potential control groups is greater if we have a longer period with which to work. With that in mind, I’ll briefly summarize the solutions we’ve proposed for the immediate, medium, and longer terms.
Immediate Solutions
Given the lack of individuals who did not pursue education or training after high school in the ELMLP data, researchers could use a “synthetic cohort approach.” The approach is “synthetic” because the comparison groups would be drawn from different data sources that contain earnings information (e.g., the Labour Force Survey or long-form census) but the cohorts are defined using the same sample selection criteria.
The key challenge here is matching, as closely as possible, the relevant characteristics such as age, gender, and industry of employment across the data sources. Many techniques for matching exist, but the specificity of the cohorts would be restricted by the limited demographic and occupational information available in ELMLP. Nevertheless, this synthetic cohort approach offers a straightforward mechanism to develop a reasonable earnings comparison group using existing datasets.
Medium-term Solutions
ELMLP links records from the Postsecondary Student Information System (PSIS) and Registered Apprenticeship Information System (RAIS) to tax records from the T1 Family File (T1FF). The T1FF data are linked only for those who exist in the other two databases. It should therefore be a relatively straightforward exercise for Statistics Canada to embed into the ELMLP data environment those individual T1FF files not already matched to a PSIS or RAIS record with a so-called “reverse data linkage.”
The reverse-linked individuals would cover all those who did not participate in a public college or university program, nor in a registered apprenticeship program, in Canada. That would include our desired target population (i.e., individuals with only high school education), as well as individuals who attended an international PSE programme. The inclusion of this latter group would risk skewing upward the earnings of the comparison group by including immigrants and returning Canadians educated outside of Canada.
One solution to this problem is to isolate the reverse-linked individuals to those with consistent earnings from ages 18 or 19 onward — a strong indication of regular work immediately following high school. Although this might exclude young parents who were temporarily out of the labour force, this would be less distortionary than including all reversed-linked records.
Longer-term Solutions
The best solution for developing a reliable and comprehensive earnings comparison group is to link the long-form census (and potentially the 2011 National Household Survey) with the existing ELMLP data environment. The long-form census provides an immense amount of detail about individuals’ education levels and earnings. Combining this linkage with the reverse-linkage of remaining T1FF records, as suggested above, would provide a clear-cut set of individuals who did not participate in post-secondary education or training.
Moving toward the development of a comprehensive earnings comparison group is essential if we want a clear picture of the earnings premium associated with education and training and how this has evolved over time.
Looking Ahead
The ELMLP data environment is a major step forward in providing extremely useful data linkages for policy-relevant research. I understand that Statistics Canada and LMIC have been working together on addressing current limitations of ELMLP via new data linkages, as well as improving the labour market indicators reported from this data environment. Developing additional linkages will further enhance the power of the data and help us better understand the multi-faceted roles that education plays in the lives of Canadians. I look forward to the innumerable insights and recommendations that this platform will provide to policymakers, educators, and Canadians across the country over the years to come.
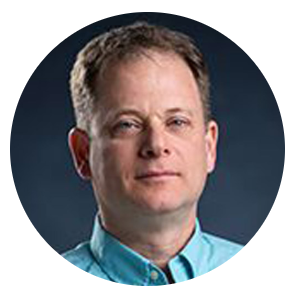
Ted McDonald is a Professor of Economics at the University of New Brunswick, the Academic Director of the New Brunswick Research Data Centre, Director of the NB Institute for Research, Data and Training (NB-IRDT) and a member of LMIC’s Labour Market Information Experts Panel.